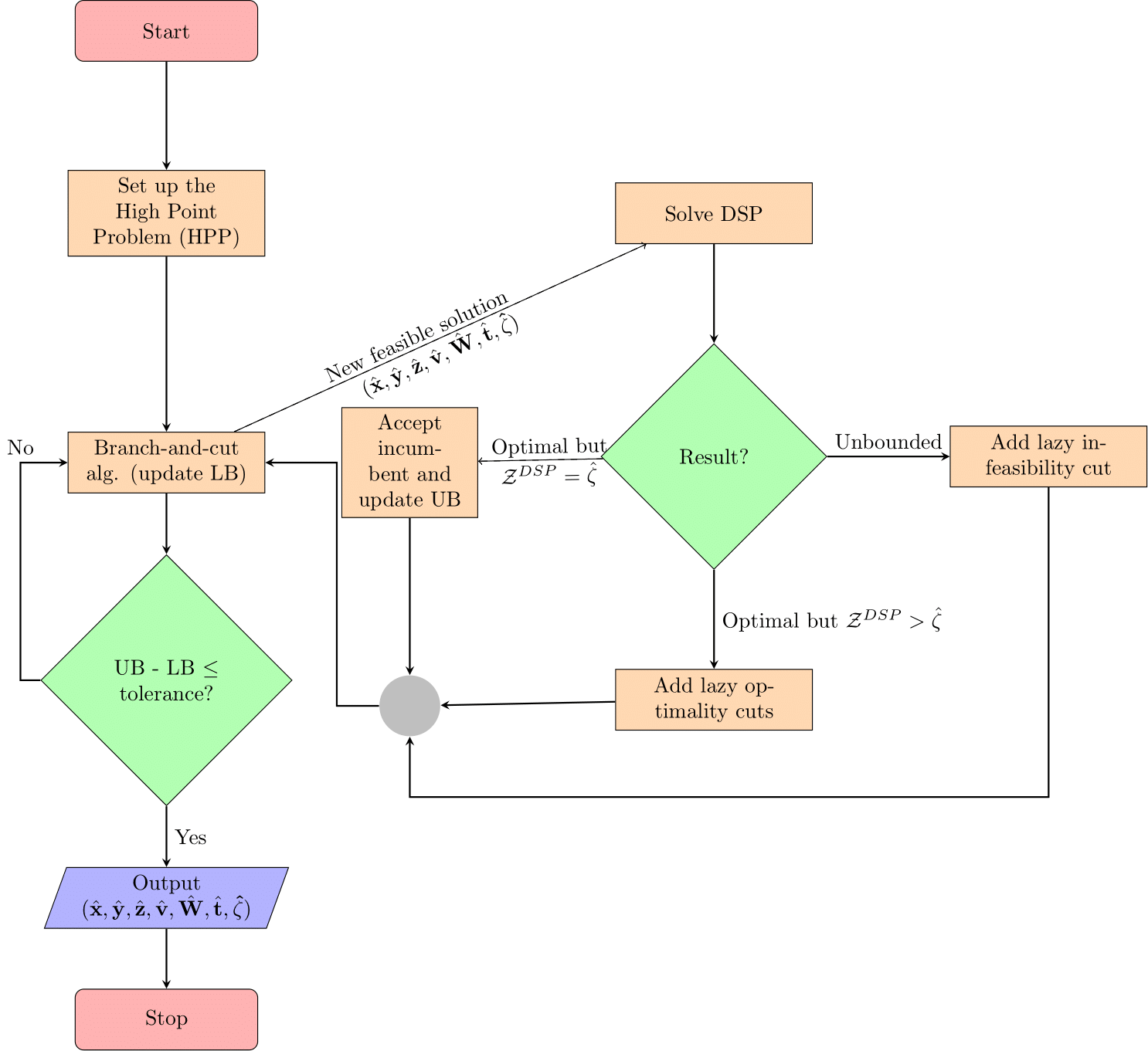
Publications
- P. Kumar and A. Khani. "An Exact Method for Solving the Bi-level transit Network Design Problem" Under review , 2022
- P. Kumar and A. Khani. "Schedule-based Transit Assignment with Online Information" Transportation Research Part C: Emerging Technologies, 2023 PDF
- X. Yu, J. Chen, P. Kumar, A. Khani, and H. Mao . "An Integrated Framework for Locating Depots in Shared Autonomous Vehicle Systems" , Transportmetrica A: Transport Science, 2022. SSRN
- P. Kumar and A. Khani. "Planning of integrated mobility-on-demand and urban transit networks." Transportation Research Part A: Policy and Practice, 2022. PDF
- P. Kumar and A. Khani. "Adaptive park-and-ride choice on time-dependent stochastic multimodal transportation network." Networks and Spatial Economics , 2021. Journal
- P. Kumar, A. Khani., E. Lind, and J. Levin. "Estimation and mitigation of epidemic risk on a public transit route using automatic passenger count data." Transportation Research Record, 2021. PDF
- P. Kumar and A. Khani. "An algorithm for integrating peer-to-peer ridesharing and schedule-based transit system for first mile/last mile access." Transportation Research Part C: Emerging Technologies, 2021. arXiv
- P. Kumar and A. Khani. "Evaluating Special Event Transit Demand: A Robust Principal Component Analysis Approach." IEEE Transactions on Intelligent Transportation Systems, 2021. PDF
- A. Webb, P. Kumar and A. Khani. "Estimation of Passenger Wait Time using Automatically Collected Transit Data." Public Transport, 2020. PDF
- P. Kumar, A. Khani, and G. Davis. "Transit Route Origin-Destination Matrix Estimation using Compressed Sensing." Transportation Research Record, 2019. PDF
- P. Kumar, A. Khani, and Q. He. "A Robust Estimation of Transit Passenger Trajectories using Automated Data." Transportation Research Part C: Emerging Technologies, 2018. PDF
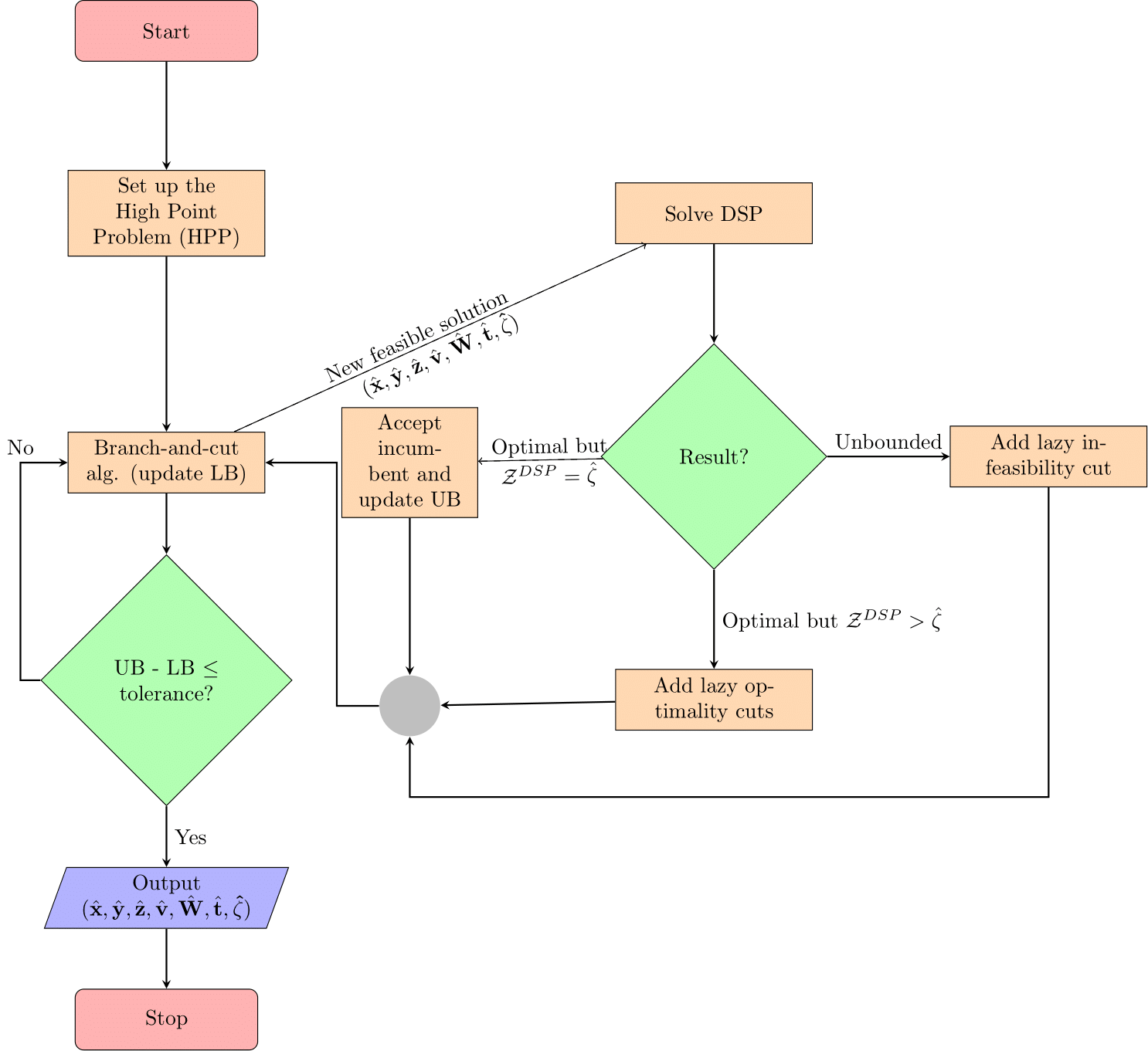
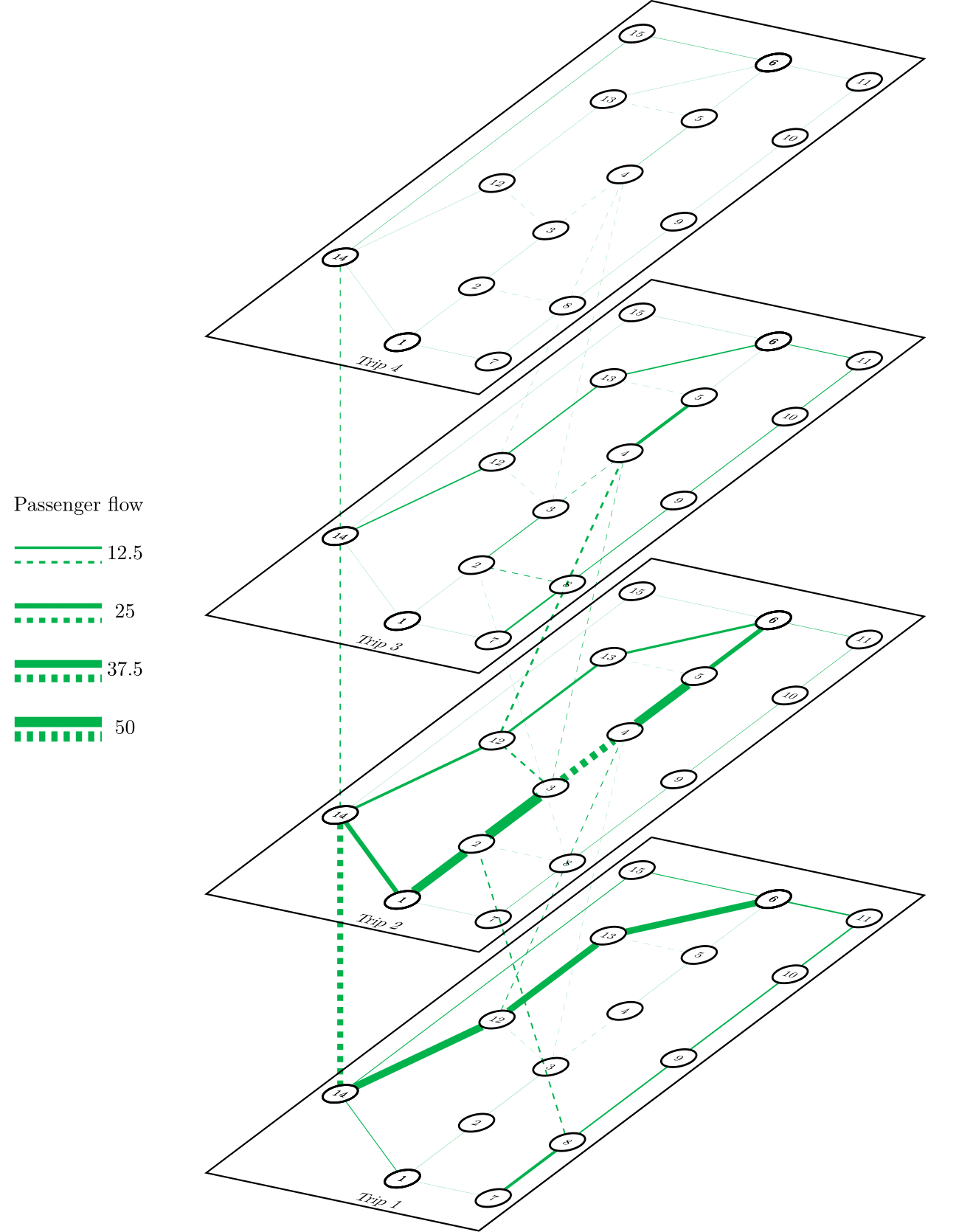
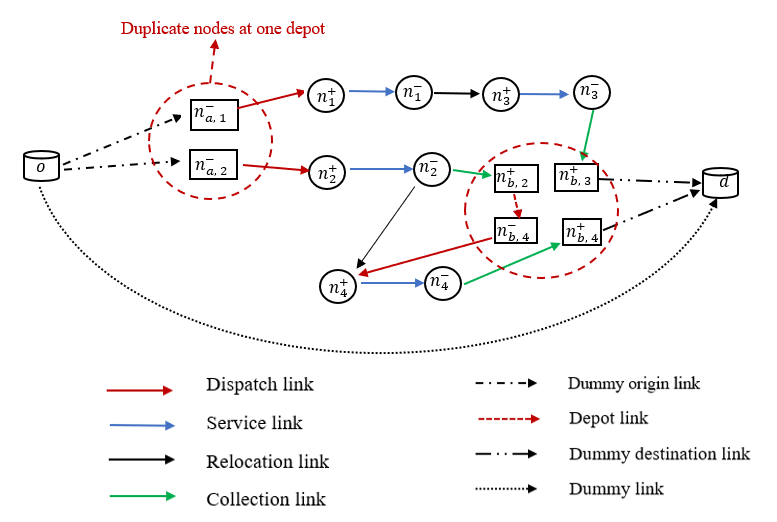
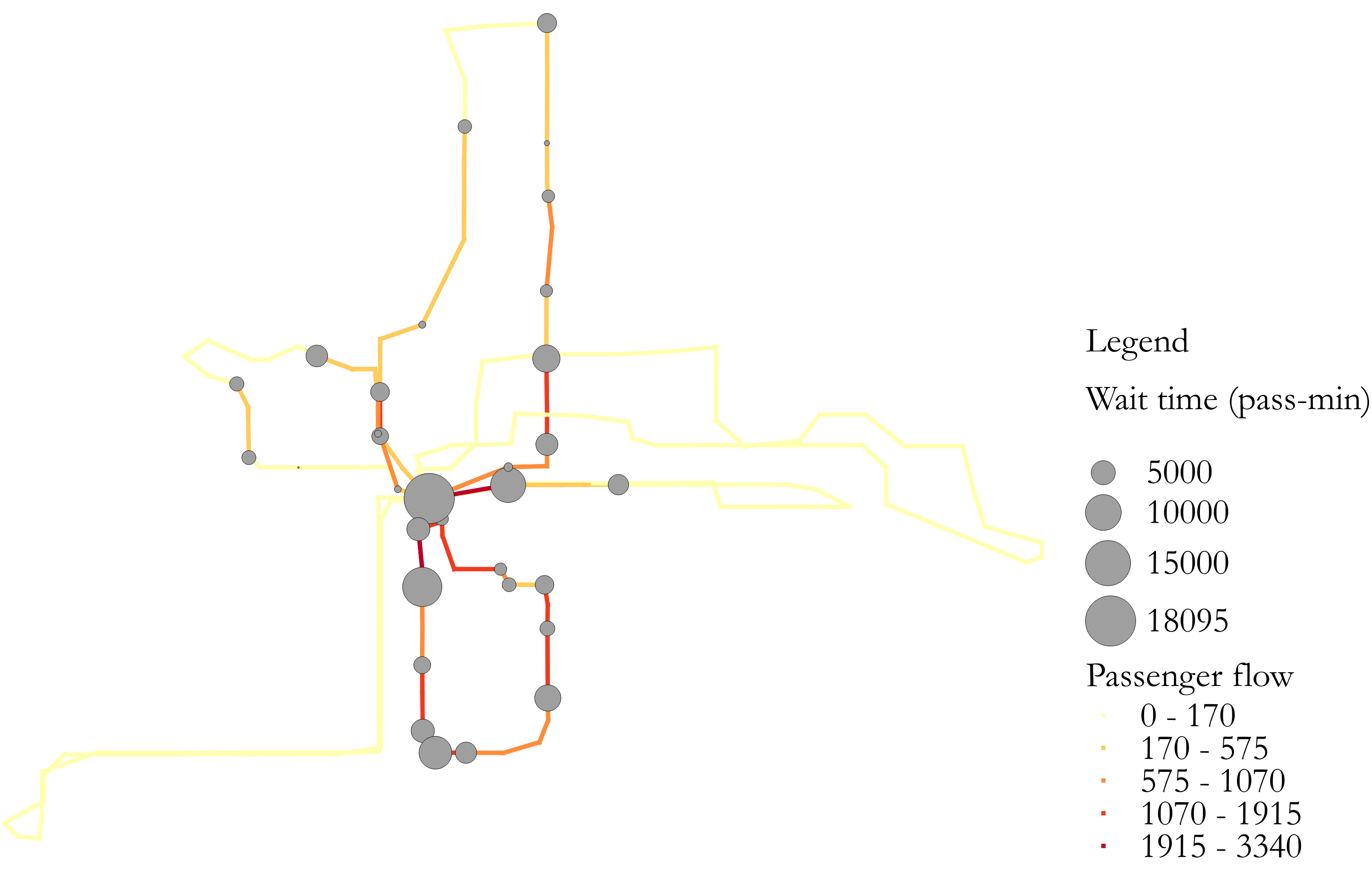
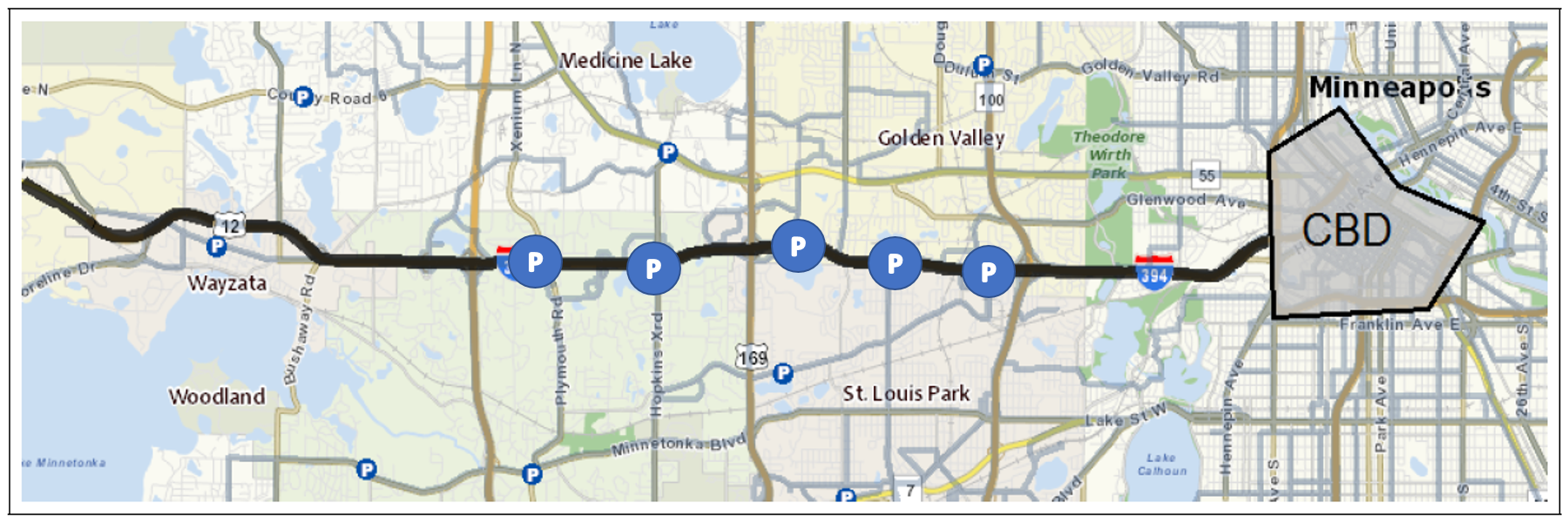

Abstract: This paper studies the potential spread of infectious disease through passenger encounters in a public transit system using automatic passenger count (APC) data. An algorithmic procedure is proposed to evaluate three different measures to quantify these encounters. The first two measures quantify the increased possibility of disease spread from passenger interaction when traveling between different origin–destination pairs. The third measure evaluates an aggregate measure quantifying the relative risk of boarding at a particular stop of the transit route. For calculating these measures, compressed sensing is employed to estimate a sparse passenger flow matrix planted in the underdetermined system of equations obtained from the APC data. Using the APC data of Route 5 in Minneapolis/St. Paul region during the COVID-19 pandemic, it was found that all three measures grow abruptly with the number of passengers on board. The passenger contact network is densely connected, which further increases the potential risk of disease transmission. To reduce the relative risk, it is proposed to restrict the number of passengers on-board and analyze the effect of this using a simulation framework. It was found that a considerable reduction in the relative risk can be achieved when the maximum number of passengers on-board is restricted below 15. To account for the reduced capacity and still maintain reasonable passenger wait times, it would then be necessary to increase the frequency of the route.

Abstract: Due to limited transit network coverage and infrequent service, suburban commuters often face the transit first mile/last mile (FMLM) problem. To deal with this, they either drive to a park-and-ride location to take transit, use carpooling, or drive directly to their destination to avoid inconvenience. Ridesharing, an emerging mode of transportation, can solve the transit first mile/last mile problem. In this setup, a driver can drive a ride-seeker to a transit station, from where the rider can take transit to her respective destination. The problem requires solving a ridesharing matching problem with the routing of riders in a multimodal transportation network. We develop a transit-based ridesharing matching algorithm to solve this problem. The method leverages the schedule-based transit shortest path to generate feasible matches and then solves a matching optimization program to find an optimal match between riders and drivers. The proposed method not only assigns an optimal driver to the rider but also assigns an optimal transit stop and a transit vehicle trip departing from that stop for the rest of the rider’s itinerary. We also introduce the application of space-time prism (STP) (the geographical area which can be reached by a traveler given the time constraints) in the context of ridesharing to reduce the computational time by reducing the network search. An algorithm to solve this problem dynamically using a rolling horizon approach is also presented. We use simulated data obtained from the activity-based travel demand model of Twin Cities, MN to show that the transit-based ridesharing can solve the FMLM problem and save a significant number of vehicle-hours spent in the system.

Abstract: The special events such as games, concerts, state fairs, etc. attract a large amount of population, which requires proper planning of transit services to meet the induced demand. Previous studies have proposed methods for estimating an average daily weekday demand, which have an inherent disadvantage in estimating the demand for a special event. We solve an idealized version of this problem i.e., we decompose a special event affected demand matrix into a regular and an outlier matrix. We start with detecting the special events in large scale transit data using the Mahalanobis distance, an outlier detection method for high dimensional data. Then, a special event demand is evaluated using state-of-the-art dimensionality reduction technique known as robust principal component analysis (RPCA), which is formulated as a convex optimization program. We show the application of the proposed method using Automatic Passenger Count (APC) data from Twin Cities, MN, USA. The methods are general and can be applied to any type of data related to the flow of passengers available with respect to time. Of practical interest, the methods are scalable to large-scale transit systems.
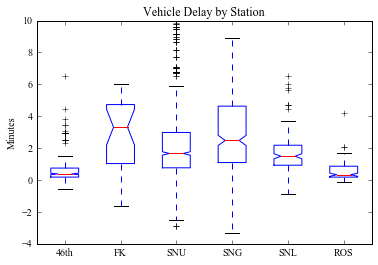
Abstract: Among the many ways to improve a transit system is a reduction in travel time as experienced by the passenger. Hence, passenger waiting times remain a topic of interest among transit planners. In this study, the effects of transit vehicle delays on passenger waiting time is investigated, as well as the effects of transfer status, boarding location, time of day, and rider travel frequency. The data used in this study were collected using automatic fare collection (AFC) and automatic vehicle location (AVL) technology. A trip chaining algorithm is used to infer the trajectory of each passenger, and as a result produce measures of passenger waiting time and vehicle delay. An analysis of an arterial Bus Rapid Transit (aBRT) line in Saint Paul, Minnesota reveals a waiting time model consistent with previous literature, a positive relationship between vehicle delay and passenger waiting time, and an insignificant relationship between transfer status and passenger waiting time. Finally, a simple model relating waiting time and vehicle delay is provided for the purpose of transit planning and waiting time estimation.
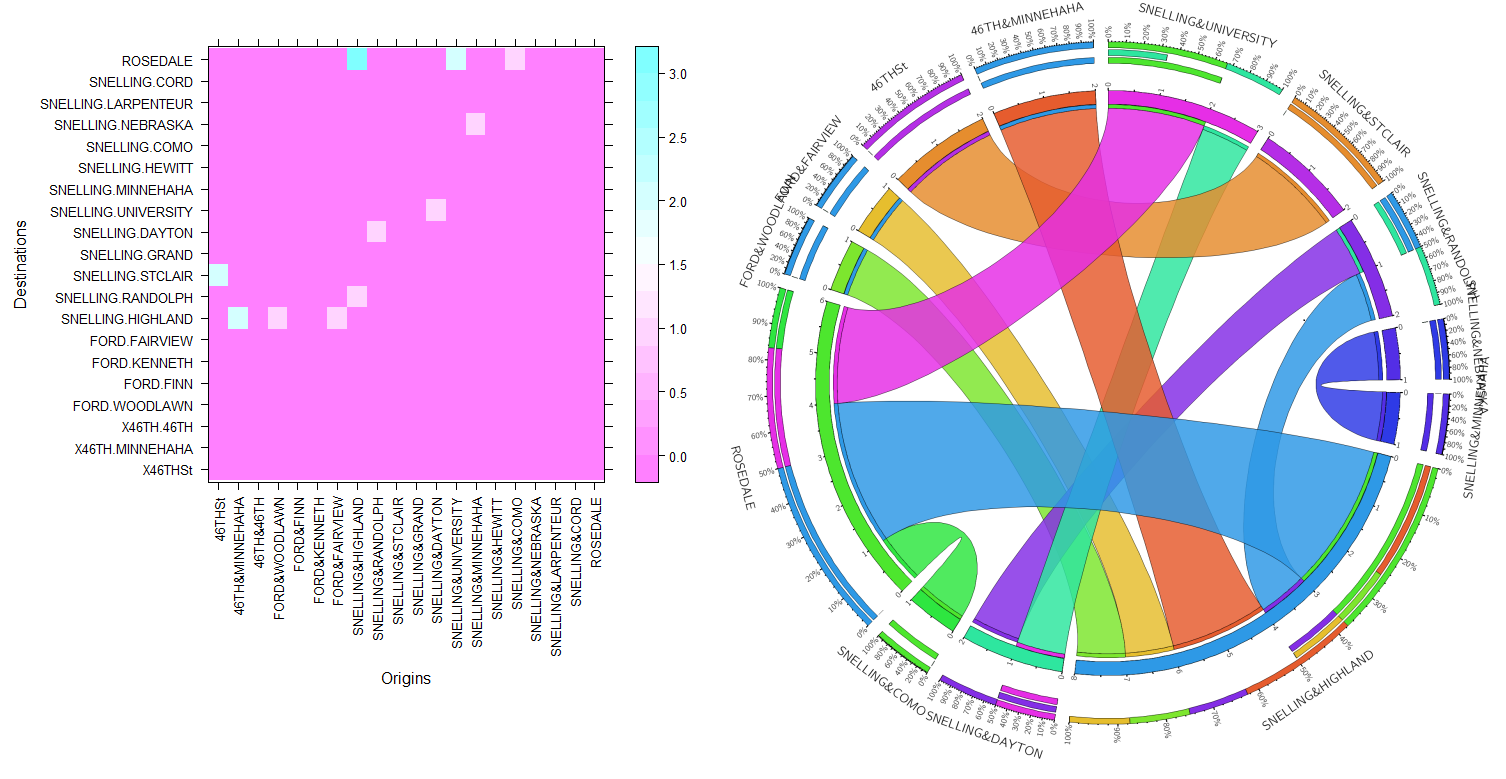
Abstract: The development of an origin–destination (OD) demand matrix is crucial for transit planning. With the help of automated data, it is possible to estimate a stop-level OD matrix. We propose a novel method for estimating transit route OD matrix using automatic passenger count (APC) data. The method uses l0 norm regularizer, which leverages the sparsity in the actual OD matrix. The technique is popularly known as compressed sensing (CS). We also discuss the mathematical properties of the proposed optimization program and the complexity of solving it.We used simulation to assess the accuracy and efficiency of the method and found that the proposed method is able to recover the actual matrix within small errors. With increased sparsity in the actual OD matrix, the solution gets closer to the actual value of the matrix. The method was found to perform more efficiently even for different demand patterns. We also present a real numerical example of OD estimation of the A Line Bus Rapid Transit (BRT) route in Twin Cities, MN.
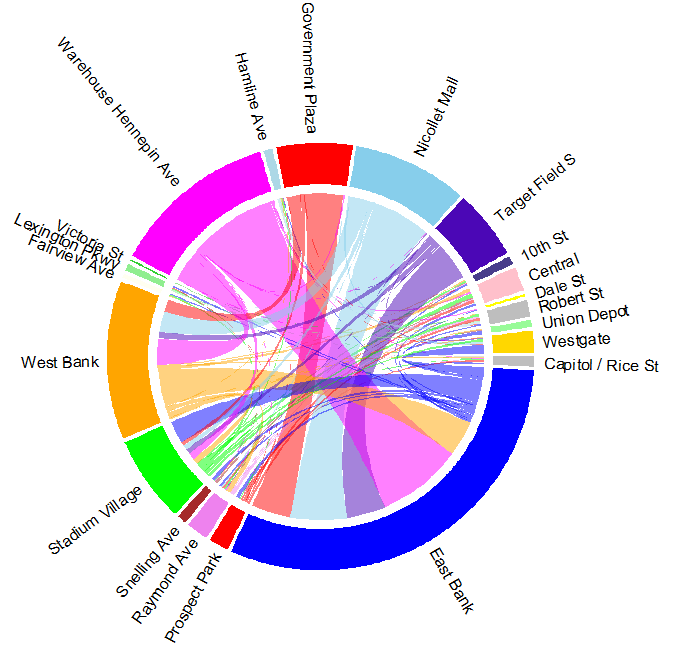
Abstract: Development of an origin-destination demand matrix is crucial for transit planning. The development process is facilitated by automated transit smart card data, making it possible to mine boarding and alighting patterns on an individual basis. This research proposes a novel trip chaining method which uses Automatic Fare Collection (AFC) and General Transit Feed Specification (GTFS) data to infer the most likely trajectory of individual transit passengers. The method relaxes the assumptions on various parameters used in the existing trip chaining algo- rithms such as transfer walking distance threshold, buffer distance for selecting the boarding location, time window for selecting the vehicle trip, etc. The method also resolves issues related to errors in GPS location recorded by AFC systems or selection of incorrect sub-route from GTFS data. The proposed trip chaining method generates a set of candidate trajectories for each AFC tag to reach the next tag, calculates the probability of each trajectory, and selects the most likely trajectory to infer the boarding and alighting stops. The method is applied to transit data from the Twin Cities, MN, which has an open transit system where passengers tap smart cards only once when boarding (or when alighting on pay-exit buses). Based on the consecutive tags of the passenger, the proposed algorithm is also modified for pay-exit cases. The method is compared to previous methods developed by the researchers and shows improvement in the number of in- ferred cases. Finally, results are visualized to understand the route ridership and geographical pattern of trips.
Doctoral Dissertation
- "Modeling and Design of Integrated Transit Systems with Strategic Passenger Behavior", 2022. PDF
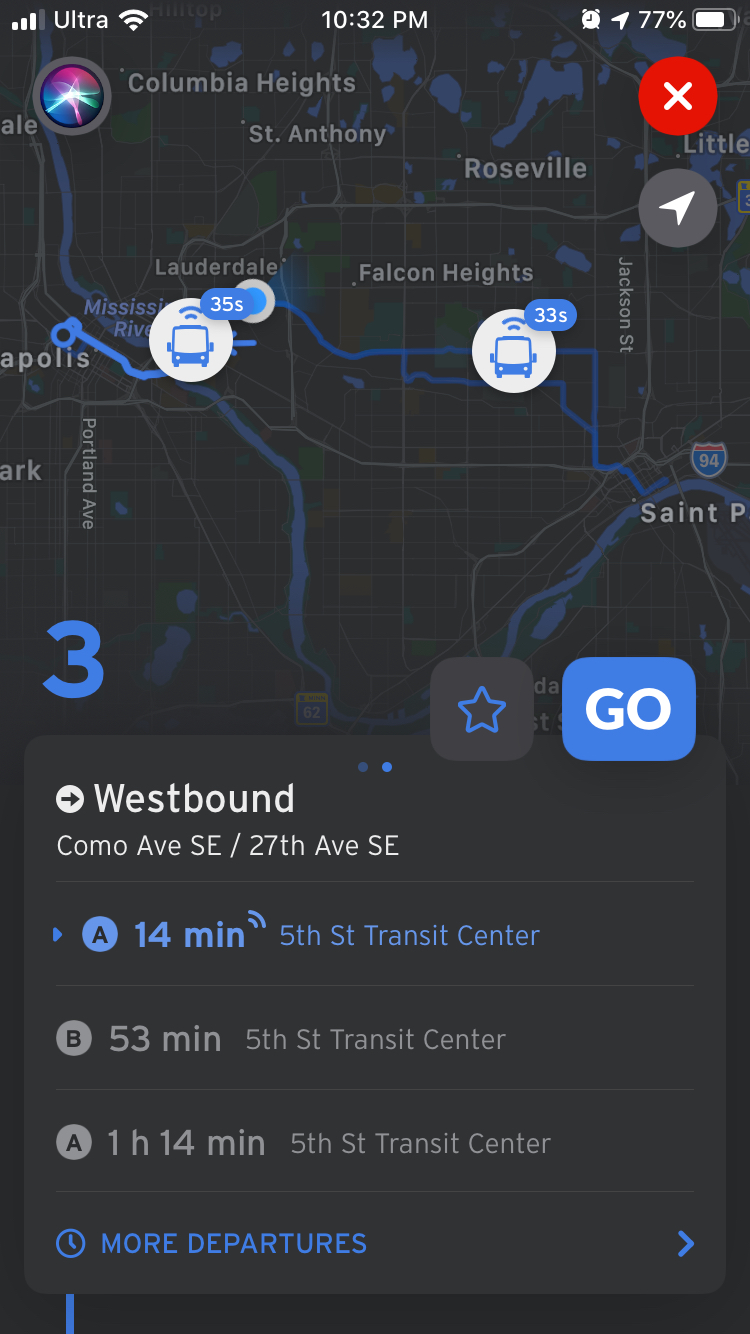
Master's Thesis
- "Transit Origin Destination Estimation using Automated Data", 2019. PDF
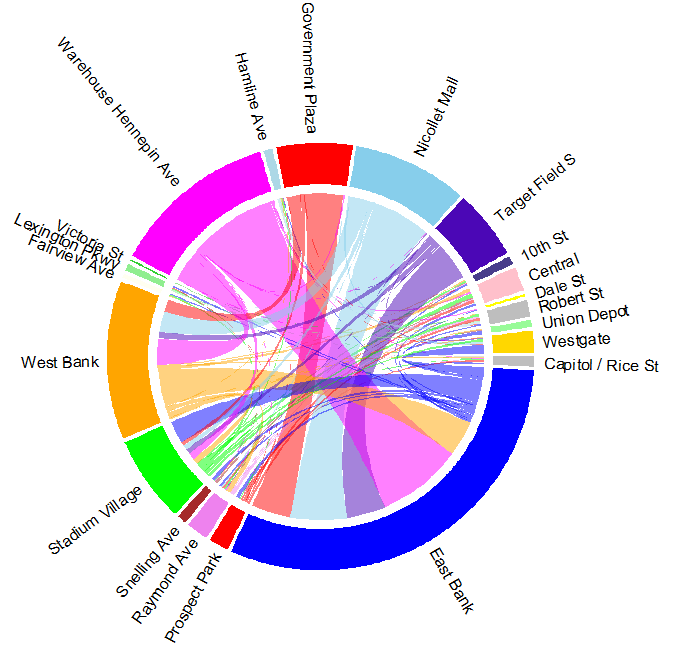
